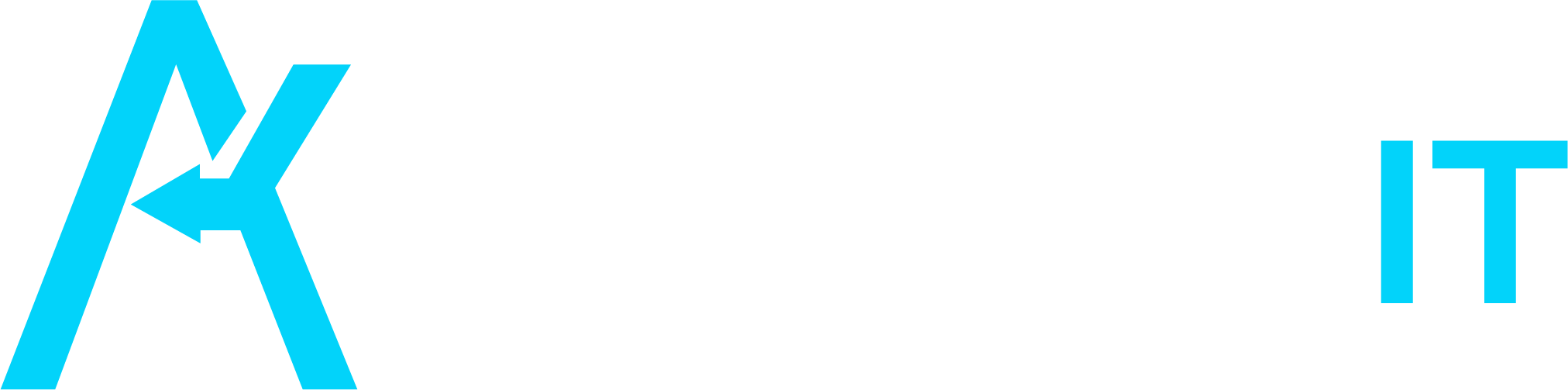
At Akross IT, we build predictive intelligence systems that help you stay ahead of the curve—whether it’s anticipating customer churn, forecasting demand, preventing equipment failure, or detecting fraud before it happens. Our solutions are tailored to your business goals, integrating seamlessly with your existing systems to turn historical data into strategic foresight.
Custom Predictive Models
We design tailored models for sales forecasting, risk scoring, churn prediction, and more, using cutting-edge machine learning algorithms.
Real-Time Forecasting
Implement predictive systems that analyze live data streams to offer dynamic forecasts and timely decision support.
Behavioral and Trend Analysis
Understand patterns in user activity, market trends, and operational metrics to uncover hidden opportunities and risks.
Automated Alerts and Recommendations
Receive intelligent notifications and suggested actions when forecasts indicate anomalies or potential problems.
Integration with Business Platforms
Connect predictions directly to your ERP, CRM, or analytics platforms to inform workflows and optimize operations.
Scalable and Adaptable Architecture
Our models grow with your business, adjusting to new data and evolving business needs for long-term predictive accuracy.
We begin by aligning predictive efforts with your key business objectives. Whether it's reducing churn, increasing operational efficiency, or improving financial planning, we define the use case clearly.
Our team then conducts a data audit to assess the quality, sources, and completeness of your historical and real-time data, including structured and unstructured formats.
We transform raw data into structured formats ready for modeling. This includes cleaning, normalization, time-series formatting, and correlation analysis.
Feature engineering is critical—we extract relevant signals, trends, and contextual information that help models understand underlying behaviors and influences.
Data enrichment techniques may also be used, incorporating third-party or external data for greater model depth.
Using a combination of regression, time-series forecasting, and ensemble learning methods (such as XGBoost, ARIMA, LSTM networks), we train and evaluate multiple models tailored to your problem.
Our data scientists tune parameters, test on historical datasets, and validate against future scenarios using techniques like cross-validation and backtesting to ensure robustness.
Before full deployment, we create simulation environments to test how predictions hold up under various scenarios. Forecasts are then visualized through intuitive dashboards that allow users to explore trends, compare “what-if” outcomes, and drill down into influencing factors.
These dashboards become part of your operational intelligence toolkit for strategic planning.
Once models are validated, we deploy them using scalable infrastructure—whether cloud-based, on-premise, or hybrid. APIs and connectors are developed to plug predictive outputs into your business systems (CRMs, ERPs, analytics platforms), so forecasts can inform real-time decisions and automated actions.
Live data flows into the predictive engine, enabling continuous updates to forecasts. When anomalies or significant trend shifts are detected, the system triggers alerts and prescribes actions—like promotional campaigns, operational changes, or resource reallocation.
This ensures decisions are timely and backed by intelligent foresight.
Predictive models are not static—they learn and improve over time. We implement feedback loops that collect outcome data (e.g., did churn occur as predicted?) and feed it back into the system for retraining.
Model drift is monitored continuously, and updates are rolled out to maintain performance as your business environment evolves.
Identify customers at risk of leaving and recommend timely interventions to retain them.
Use historical data and seasonal patterns to predict future revenue streams and set realistic sales targets.
Forecast equipment failures and schedule maintenance proactively, reducing downtime and saving costs.
Anticipate credit defaults, market volatility, or fraud attempts with AI-enhanced financial models.
Improve supply chain efficiency by predicting product demand, optimizing stock levels, and minimizing waste.